EMBRIODEEP
Development of algorithms based on Deep Learning for the improvement of embryo selection using artificial vision technologies and its application in a prototype demonstrator for its exploitation in the healthcare field.
To develop a system of assistance for embryo selection using Deep Learning algorithms and the most robust artificial vision technology applied in a non-invasive clinical protocol for embryo selection, which automatically analyses: morphokinetic variables, variables related to genomics and proteomics, variables related to oxidative stress, other variables intrinsic to the assisted reproduction cycles, extrinsic variables resulting from the working environment and the historical health records of all the births cultivated in Time Lapse.
Taking into account all the data from these variables, a predictive model of successful implantation will be obtained and patterns will be investigated to enable the development of an alert system to detect and simulate in silico situations in which an embryo is more likely to achieve successful implantation. These alerts will form part of the care support system for the selection of the best embryo and will facilitate decision-making in an assisted reproduction centre.
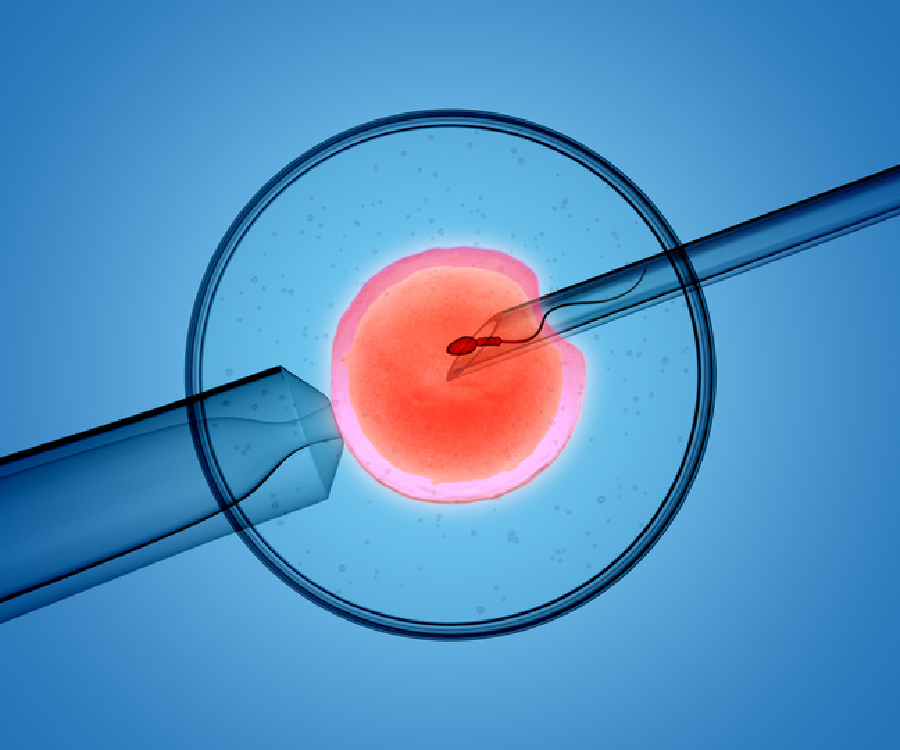
This project arises from the concern of the IVI Group’s parent company for the need to implement personalised clinical protocols that help to increase the success of each assisted reproduction cycle and also produce a reduction in the number of attempts made until reproductive success is achieved. The aim is to incorporate digitalisation technologies” through the development of services and models of advanced analytics and data visualisation that help in decision making, specifically with regard to competent embryos and critical points in the process.
Currently, there is very limited work using Artificial Intelligence (AI) and computer vision techniques to assess which embryos are most likely to have a successful final implantation. This project will provide a more robust computer vision technology framed in a non-invasive clinical protocol that allows in silico monitoring and evaluation of embryo growth and implantation success with Deep Learning; optimising processes and their performance through computer vision technologies and the development of algorithms based on Deep Learning.
Therefore, the establishment of a new, more robust machine vision technology framed in a non-invasive clinical protocol capable of automatically selecting the embryo most likely to implant from a cohort while alerting of risk situations, would mean a reduction in the time spent by embryologists on data evaluation during laboratory procedures. It would also reduce inter-observer variability.
The expected results are important because of the different family projects (heteroparental, homoparental, single-parent families), the delay in childbearing, the increase in obesity, and many other factors have increased the demand for more personalised assisted reproduction services and led to a change in the value chain approach of assisted reproduction clinics.
A better, more successful and personalised service is expected to be an attraction for potential patients and a very important competitive advantage that will result in an increase in the number of patients and an increase in turnover.
The classical method based on spot observation by experts has a number of limitations:
– It is a subjective assessment.
– The assessment is made at discrete times, based on point-in-time observation.
– There are negative effects of manipulation of the culture environment.
– The classification of embryos is divided into 4 categories defined by ASEBIR (Association for the Study of Reproductive Biology).
Faced with these limitations, artificial vision techniques and Deep Learning algorithms can mitigate or minimise them, leading to an assessment method with the following characteristics:
– Obtaining objective quantitative parameters.
– Faster evaluation of embryos.
– Alarm system that allows early identification during embryo culture.
– Provides automated information on a wide variety of parameters (morphokinetic, proteomic, genomic, oxidative stress, etc.) to facilitate decision-making by embryologists.
Therefore, the aim of this project is to solve the limitations of the aforementioned research by developing a new system based on Deep Learning algorithms and artificial vision technologies that automatically analyse a wide range of parameters that affect the success of implantation. With all this, it will be possible to develop a new diagnostic method that will facilitate decision-making by embryologists, increasing the success of embryo implantation and, consequently, increasing the success of the assisted reproduction treatments offered by the entity. In addition, the aim is to develop an alert system that allows the early identification of anomalous or suboptimal values in previously identified parameters of interest with respect to the success of implantation. The satisfactory achievement of this project would mean the clinical application of a new protocol for embryo identification and selection, as well as an associated alert system, which will substantially improve the effectiveness of the technique and, consequently, the reproductive success of the treatments offered by the entity.
In this sense, since the incorporation of the technology targeted by this project will enable greater control and better monitoring and follow-up of the process of other centres, and will allow us to identify the relationship between specific problems or inefficiencies, it will be an important attraction for our suppliers or other companies in the pharmaceutical sector or in the development of healthcare equipment with a view to carrying out controlled clinical trials or for the validation of new products or technologies.
Partners
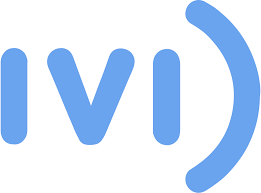
